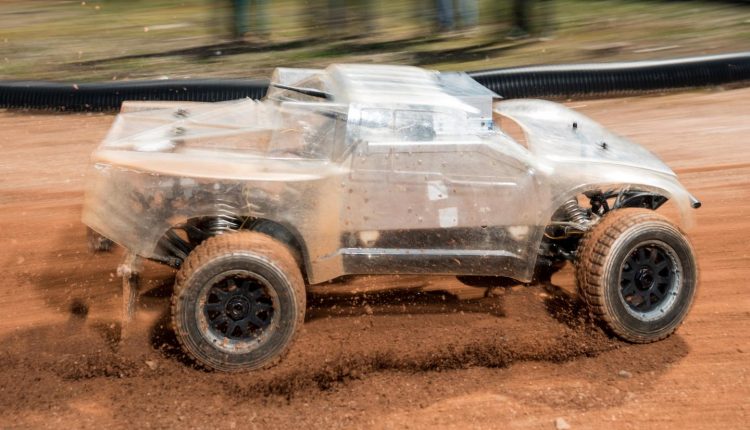
How these driverless cars stay in control under hazardous conditions
With many automotive manufacturers testing autonomous driving technology on ordinary roads, a team of researchers from the Georgia Institute of Technology decided to figure out a way to keep these vehicles from getting out of control when faced with hazardous conditions.
The team devised a new way to help keep a driverless vehicle under control as it maneuvered to the edge of its handling limits, which could impact the safety of future self-driving cars.
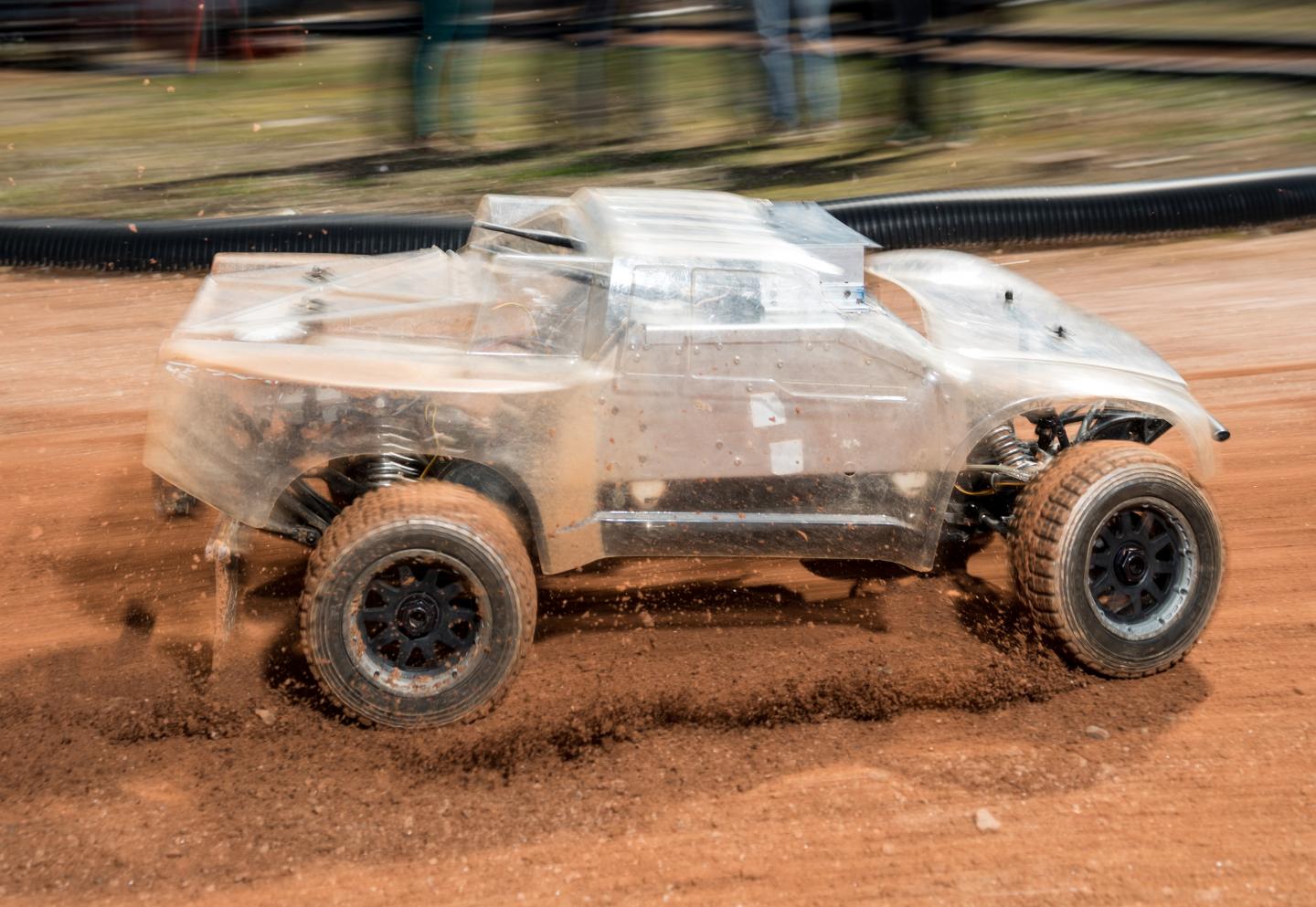
They then tested their technology by racing, sliding, and jumping one-fifth-scale, fully autonomous auto-rally cars at speeds equivalent to 90 mph.
How it works
The technique uses advanced algorithms and on-board computing, along with sensing devices, to increase vehicular stability while maintaining performance.
“An autonomous vehicle should be able to handle any condition, not just drive on the highway under normal conditions,” said Panagiotis Tsiotras, an AE professor who is an expert on the mathematics behind rally-car racing control. “One of our principal goals is to infuse some of the expert techniques of human drivers into the brains of these autonomous vehicles.”
When it comes to traditional self-driving cars, they tend approach driving in the same way whether they’re on a straight, smooth path or at the edge of a roadway. The new method, called model predictive path integral control (MPPI), was developed to address these non-linear dynamics involved in controlling a vehicle near its friction limits.
Researchers from Georgia Tech’s Daniel Guggenheim School of Aerospace Engineering (AE) and the School of Interactive Computing (IC) have assessed the new technology
The work, tested at the Georgia Tech Autonomous Racing Facility, is sponsored by the U.S. Army Research Office. A paper covering this research was presented at the recent International Conference on Robotics and Automation (ICRA), held May 16-21.
The team implemented statistics when dealing with the large amounts of handling-related information, along with data regarding the dynamics of the vehicular system, to compute the most stable trajectories from a slew of possibilities.
The MPPI control algorithm was processed by the high-power graphics processing unit (GPU) embedded in the vehicle to continuously carry sample data coming from GPS hardware, inertial motion sensors, and other sensors. The on-board hardware-software system performs real-time analysis of a vast number of possible trajectories and then informs the vehicle on the best handling decisions moment-by-moment.
Previously, optimal- control data inputs could not be processed in real time, so this technology is one of the first to carry out this type of computing.
The researchers custom built two 48-lb. auto-rally vehicles using special electric motors to achieve the right balance between weight and power. The cars included a motherboard with a quad-core processor, a potent GPU, and a battery.
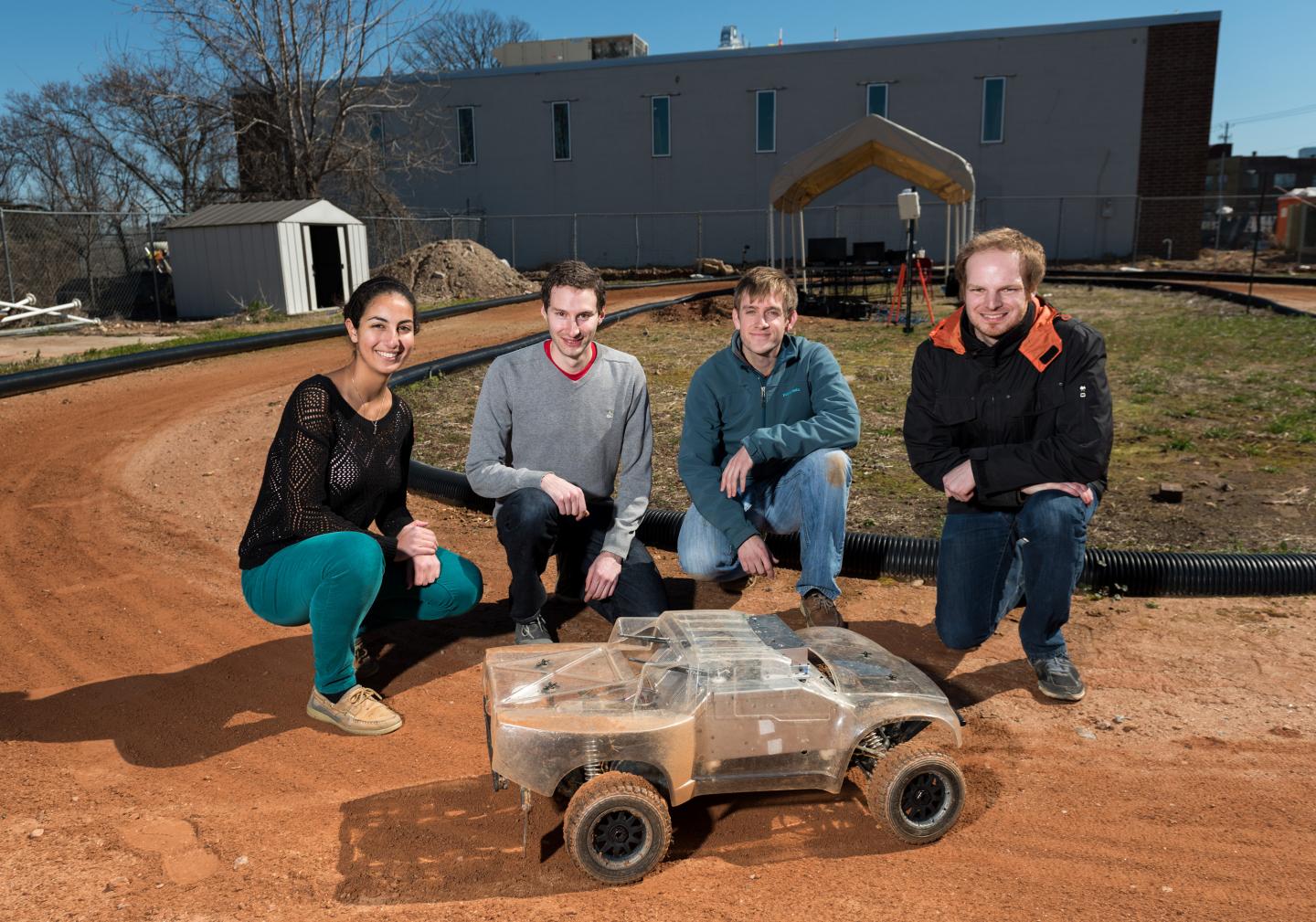
Each vehicle was also given two forward-facing cameras, an inertial measurement unit, and a GPS receiver, along with sophisticated wheel-speed sensors. The power, navigation, and computation equipment was contained in a rugged aluminum enclosure so that it would be able to withstand violent rollovers.
These rolling robots were designed to test the team’s control algorithms without any need for off-vehicle devices or computation, other than a nearby GPS receiver. The on-board GPU lets the MPPI algorithm sample more than 2,500, 2.5-second-long trajectories in under 1/60 of a second.
An important part of the new autonomous approach revolved around three key elements of system functionality: staying on the track, achieving a desired velocity, and the control system overall.
An important aspect in the team’s autonomous-control approach centers on the concept of “costs” – key elements of system functionality. Several cost components must be carefully matched to achieve optimal performance.
By balancing these factors. the team could get a robotic vehicle to maximize speed while staying under control, according James Rehg, a professor in the Georgia Tech School of Interactive Computing.
Comments are closed, but trackbacks and pingbacks are open.