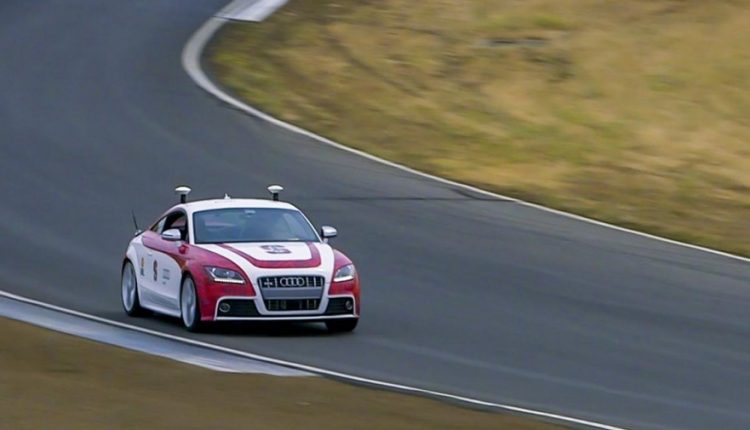
Neural Network Integrates Driving Experience to Make Autonomous Cars Safer
By Ruth Seeley
Using artificial intelligence to build a neural network that can integrate past driving experiences and physics-based trajectories, autonomous cars will be able to fine-tune their in-the-moment environmental assessments and benefit from past driving experiences to become more flexible, responsive, and safer, especially in extreme conditions.
At least that’s the hope of mechanical engineering students at Stanford, who tested their system on a California racetrack and at a winter test facility using two autonomous vehicles, a Volkswagen GTI and an Audi TTS.
Control systems for autonomous cars need access to information about the available road-tire friction. This information dictates the limits of how hard the car can brake, accelerate and steer in order to stay on the road in critical emergency scenarios. If engineers want to safely push an autonomous car to its limits, such as having it plan an emergency maneuver on ice, they have to provide it with details, like the road-tire friction, in advance. This is difficult in the real world where friction is variable and hard to predict.
“With so many self-driving cars on the roads and in development, there is an abundance of data being generated from all kinds of driving scenarios,” said Nathan Spielberg, a graduate student in mechanical engineering at Stanford and lead author of the paper about this research, published March 27 in Science Robotics. “We wanted to build a neural network because there should be some way to make use of that data. If we can develop vehicles that have seen thousands of times more interactions than we have, we can hopefully make them safer.”
The group ran comparison tests for their new system at a racetrack. Using the autonomous Audi, the car was controlled by the physics-based autonomous system, pre-loaded with set information about the course and conditions. When compared on the same course during 10 consecutive trials, the autonomous Audi and a skilled amateur driver generated comparable lap times. The neural network was then loaded into the autonomous Volkswagen, which performed similarly running both the learned and physics-based systems, even though the neural network lacked explicit information about road friction.
In simulated tests, the neural network system outperformed the physics-based system in both high-friction and low-friction scenarios. It did particularly well in scenarios that mixed those two conditions.
Source: Stanford University