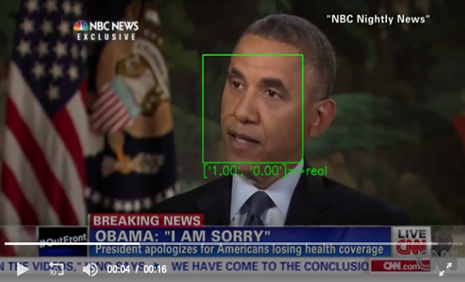
Defeating Deepfake Detectors
Systems that detect deepfakes, videos that manipulate real-life footage via artificial intelligence, can now be deceived. Computer scientists demonstrated how it can happen at the WACV 2021 conference online Jan. 5 to 9, 2021.
Detectors are defeated by adding inputs, called adversarial examples, into each video frame. The adversarial examples cause AI systems machine learning models to make mistakes. The attack continues to work after videos are compressed.
Shehzeen Hussain, a UC San Diego computer engineering Ph.D. student and first co-author on the WACV paper, indicated that this is a real threat. Robust adversarial deepfakes can be crafted even when an adversary may not be aware of the inner workings of the machine learning model used by the detector.
In deepfakes, a subject’s face is modified to create convincingly realistic footage of events that never actually happened. Typical deepfake detectors focus on the face in videos: tracking it and passing on the cropped face data to a neural network that determines whether it is real or fake. State-of-the-art deepfake detectors rely on machine learning models to identify fake videos.
When attackers have knowledge of the detection system, they can design inputs to target the blind spots of the detector and bypass it, according to Paarth Neekhara, the paper’s other first co-author and a UC San Diego computer science student.
The team tested their attacks using two scenarios, and both were highly successful. The team also declined to release their code so it wouldn’t be used by hostile parties.
To improve detectors, researchers recommend an approach similar to what is known as adversarial training, whereby an adaptive adversary continues to generate new deepfakes that bypass the current state-of-the-art detector, while the detector continues improving in order to detect the new deepfakes.