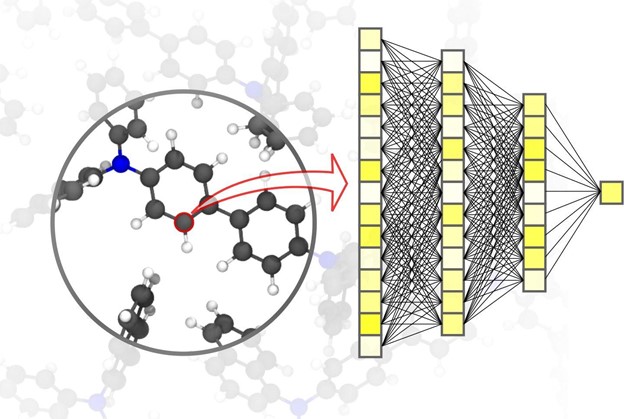
Machine learning aids in fast and accurate material science simulations
Novel materials development depends heavily on the availability of fast and accurate simulation methods. Machine learning is on its way to enabling researchers to innovate complex material systems in a completely virtual environment. The method was recently published in the Nature Materials journal, by a researcher from Karlsruhe Institute of Technology (KIT) and his colleagues from Göttingen and Toronto.
The production of novel materials is beneficial for many diverse applications, including renewable energies and new medicines. AI subset, machine learning, takes simulations in material sciences to the next level. The use of neural networks specifically tailored to material simulations enables a significant speed advantage, according to physicist and AI expert Professor Pascal Friederich, Head of the AiMat – Artificial Intelligence for Materials Sciences research group at KIT’s Institute of Theoretical Informatics (ITI).
In an article published in Nature Materials, Pascal Friederich, who is also associate group leader of the Nanomaterials by Information-Guided Design division at KIT’s Institute of Nanotechnology (INT), presents an overview of the basic principles of machine learning used for simulations in material sciences. This also includes the data acquisition process and active learning methods. For simulations in materials science, it is important to achieve high accuracy over different time and size scales, ranging from the atom to the material, while limiting computational costs. In the article, the team discusses current applications including small organic molecules and large biomolecules, structurally disordered solid, liquid, and gaseous materials, and complex crystalline systems.
The researchers suggest the development of hybrid methods that combine machine learning (ML) and molecular mechanics (MM) methods. MM simulations use force fields to calculate forces acting on each individual particle and thus predict motions. The potentials of the ML and MM methods are similar, a tight integration with variable transition areas is possible. The hybrid methods could significantly accelerate the simulation of large biomolecules or enzymatic reactions in the future.
Original Release: Eureka Alert